Low Birth Weight Classification With Synthetic Minority Over Sampling Technique Random Forest
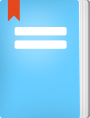
Low Birth Weight Classification With Synthetic Minority Over Sampling Technique Random Forest
Poltekkes Kemenkes Yogyakarta
2023-10-30
eng
info:eu-repo/semantics/article
application/pdf
Low birth weight (LBW) is defined as a condition where the birth weight is less than 2500 grams. Infants born with LBW conditions are more susceptible to disease and have a higher risk of dying at an early age. LBW conditions that are prone to unbalanced data can be classified using the Synthetic Minority Oversampling Technique (SMOTE) random forest method. The analysis was processed on the 2017 Indonesian Demographic and Health Survey (IDHS) data to identify important variables in predicting the incidence of LBW. The results showed that the SMOTE random forest model provided an accuracy value of 79.84%, sensitivity of 30.99%, specificity of 83.6%, and AUC of 62%. Important variables in predicting the incidence of LBW were the number of antenatal care visits, wealth quantile, maternal age at delivery, iron supplementation, marital status, and twins’ birth.